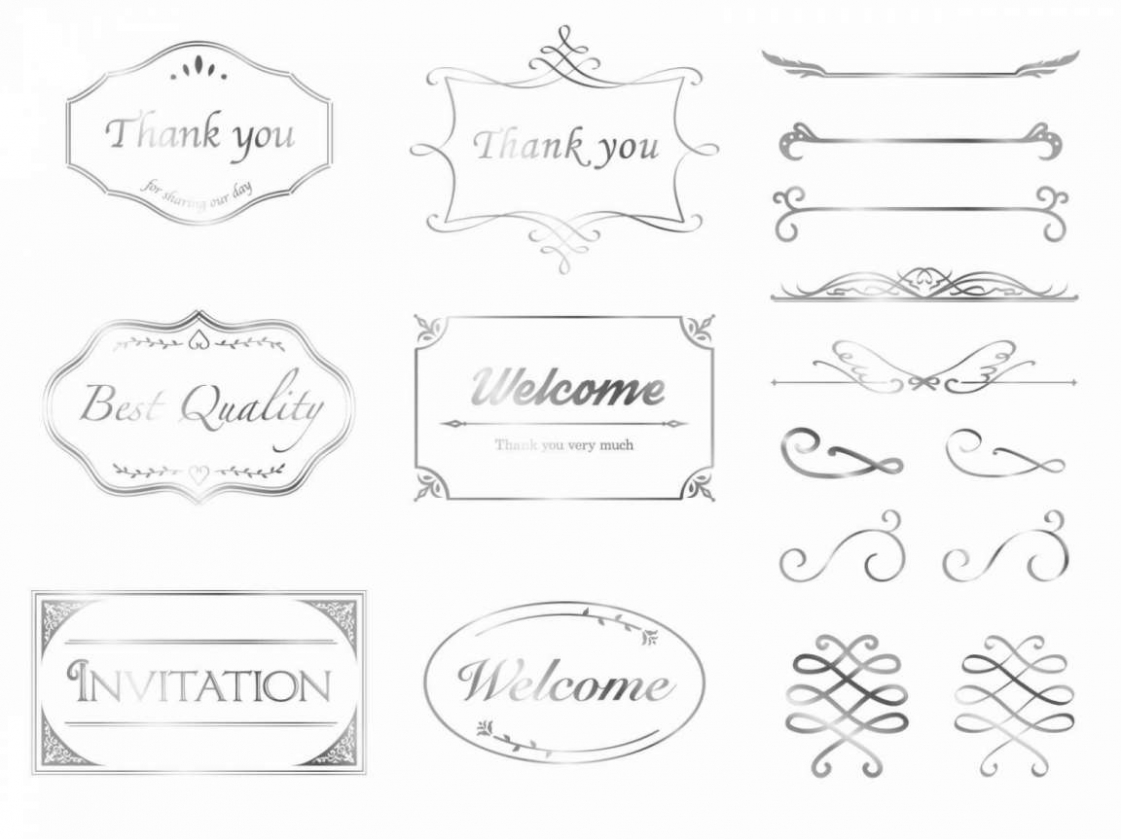
ロゴやマークのデザインをしていると、どうもアイデアが出てこないということはないでしょうか。私もデザイナー時代には煮詰まってしまうことがときどきありました。一旦迷路に入ってしまうと何を作ってもひどいものしかできません。
しかし、クライアントへの納期は迫ってきます。そのようなときの気持ちの切り替え方やアイデアの見つけ方など、対処法について個人的な体験から紹介していきます。
対処法1「クライアントの好みを分析してみる」
アイデアに煮詰まったときは、クライアントの好みや求めているものをしっかり分析してみましょう。一度提案したものの、クライアントがまったく納得していない、むしろ難色を示してきたというときには特に重要です。まず、ロゴやマークを使用する会社や店舗、施設について「どのようなコンセプトなのか」という点を中心にもう一度じっくり考えてみます。
会社や医療機関であれば経営理念や指針を参考にするといいでしょう。公式サイトがある場合は、代表挨拶からヒントが得られることもあります。しかし、中にはコンセプトが定まっていないというケースも出てきます。
デザインを提案するたびに考えが二転三転するというクライアントも珍しくはありません。
これは、デザインする側にとっても非常に困るケースです。ただ、このようなタイプのクライアントは、周囲の意見に流されやすかったり業種とは違う何か他のこだわりを持っていたりすることもあります。クライアントが何をもとに考えを変えてしまうのか、本当はどのようなものを求めているのか、趣味や個人的なこだわり、好みの傾向などを分析し直してみましょう。
対処法2「同業他社のロゴやマークからヒントを得る」
ロゴやマークを作成するとき、同じ業種の他社のデザインを一通り見ておくことは非常に重要です。これは、知らずに類似したデザインを作ることでトラブルに発展することを防ぐうえでも欠かすことはできません。
特に酷似したデザインとなると、訴訟に発展するケースも出てきます。たとえクライアントが気に入ってもらい納品が済んだ後であっても、契約次第ではデザイナーも何らかの責任を問われることもあります。大きな企業になれば、ロゴやマークはすべて商標登録をすることも視野に入れて作成しましょう。
同業他社のロゴやマークを見ることは、類似デザインを回避できるだけではありません。その中から新たなヒントを得ることにも役立ちます。特に、当たり障りのないデザインを求めているクライアントの場合は有効的な対処法です。
「類似してはいないけれど傾向は似ている」という線で提案すると、安心してもらうことができ、意外と早くまとまることもあります。例えば、歯科医院なら歯をモチーフにしたデザインで個性を出すという具合です。同業他社のデザインを参考にすることは、デザイナーにとってもよい勉強にもなります。
対処法3「異業種のロゴデザインを参考にしてみる」
業種に合ったデザインを提案することは大切ですが、中には「異色な会社」や「他とは違う奇抜な店舗」を目指しているクライアントもいます。なかなか納得してもらえるアイデアが出ないときは、違う業種のロゴやマークからヒントを得てみましょう。
例えば、建設会社や電気工事会社なら「安全」や「信頼」などをアピールするのが一般的です。
しかし、クライアントが求めているイメージが「親しみやすさ」や「元気な会社」だったらどうでしょう。どこまでいっても納得してもらえるデザインは提案できません。このように、業界の本来のイメージとはやや離れたものを目指しているクライアントには、まったく違う業種のデザインからヒントを得た方が納得してもらえることもあります。
結果的に多くの人の目を引き、注目されれば成功というわけです。ただし、中には業界にとってタブーな色調や表現もありますから、その辺は説明して納得してもらいましょう。世間からの反発を買うことがあっては、意味がありません。
また、いくらクライアントの要望でも、あまりに常識を逸脱してしまうとデザイナーの実績に傷をつけることにもなります。
対処法4「ロゴやマークのデザイン集や本からアイデアを練る」
デザインに関する書籍にはさまざまものが出ています。広告や名刺、飲食店のメニュー、看板、そしてロゴやマークも多数の書籍があります。デザイナーにとって、これらの書籍は参考書のようなものです。特にデザインを始めたばかりの人は、何冊かそろえておくと役立ちます。
さまざまなロゴやマークを集めたデザイン集は、新しいものでなければ参考にならないということはありません。もちろん、トレンドを知るうえでは必要です。しかし、アイデアに煮詰まったときは古いデザイン集の方がよいヒントを得られることもあります。
10年前または20年前の書籍の中には、参考になるデザインを多数見つけることもできます。なぜなら、流行とは繰り返すものだからです。そして、もう一つ理由があげられます。それは、人は目新しいものを見ると新鮮な印象を受けるということです。
普段見慣れているデザインとはやや違うものを提案することで、興味を引かせることは十分可能です。ただし、古いものをそのまま参考にするだけでは面白いものはできません。できればその中にトレンドを加えるようにしましょう。
対処法5「一旦仕事から離れて頭の中をリセットする」
どうしてもよいアイデアが湧かないというときは、一旦その仕事から離れることも必要です。納期までの時間が少ないと、どうしても自分を追い込んでしまう人もいるでしょう。しかし、その方がますます煮詰まることもあります。
そうなれば、時間が無駄になるばかりでよい仕事はできません。煮詰まったときにはまったく違うことに目を向け、頭の中を一度リセットしましょう。近所を散策するのもいいですし、家族や友人と遊びに行くのもよいリフレッシュになります。
デザインの仕事をしていると、どうしても睡眠時間が不規則になったり徹夜作業になったりすることも多くなります。睡眠不足が続いているときはじっくり眠ることも対処法の一つです。
心身の疲れを取り、頭の中をフラットな状態にすると、意外にもすっとよいアイデアが出てくることもあります。
アイデアに煮詰まったらそのまま作業を続けないことがポイント
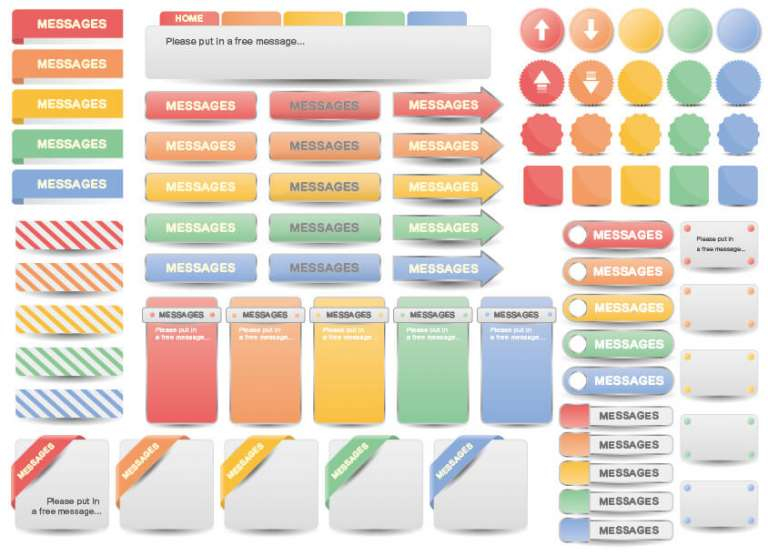
デザインの仕事をしていると、どうしてもアイデアが出てこない、クライアントに納得してもらえないという場面は出てきます。そのようなときは一度気持ちを切り替えてしまうことが一番だと思います。そのまま作業を続けても結果としてよい仕事はできません。
アイデアに煮詰まったときは古いものからヒントを得たり他社のデザインを参考にするなどしながら、クライアントをうならせるようなロゴやマークを提案しましょう。